Computer-Aided Drug Design Methods An update PMC
Table Of Content
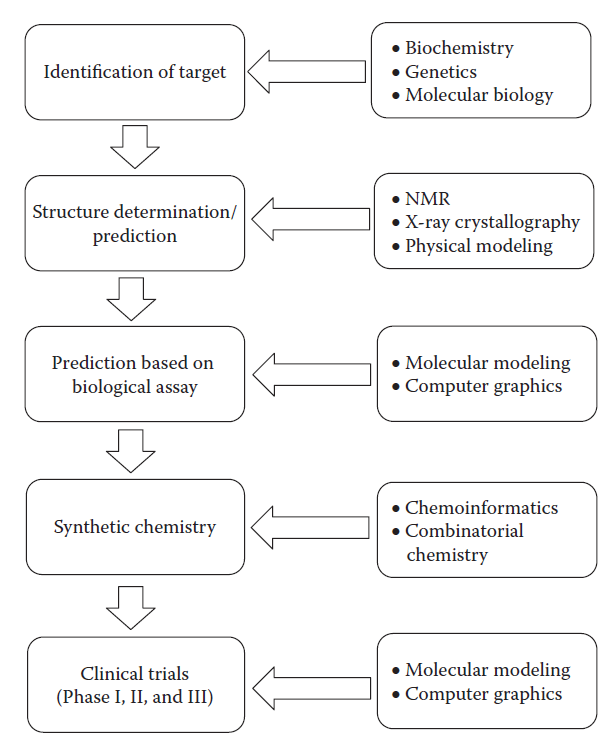
We should emphasize here that scoring functions in fast-docking algorithms and ML models are primarily designed and trained to effectively separate potential target binders from non-binders, although they are not very accurate in predictions of binding affinities or potencies. Although these methods are much slower, utilization of GPU accelerated calculations28 holds the potential for their broader application in post-processing in virtual screening campaigns to further enrich the hit rates for high-affinity candidates (Fig. 2), as well as in lead optimization stages. LBDD offers a general approach for elucidating relationships between the structural and physicochemical properties of compounds/ligands and their biological activities.
Reflecting our Progress we made as Pharmaceutical Industry
Moreover, if the physics-based and data-based scoring functions are relatively independent and both generate enrichment in the selected focused libraries, their combination can reduce the false-positive rates and improve the quality of the hits. This synergy is reflected in the latest 3DR Grand Challenge 4 results for ligand IC50 predictions59, in which the top methods that used a combination of both physics-based and ML scoring outperformed those that did not use ML. Going forward, thorough benchmarking of physics-based, ML and hybrid approaches will be a key focus of a new Critical Assessment of Computational Hit-finding Experiments (CACHE), which will assess five specific scenarios relevant to practical hit and lead discovery and optimization97. This powerful physical technique is used to predict the positions of each atom in a molecular system with respect to time which is based on Newton's laws of motions governing interatomic interactions [56]. The forces between interacting atoms are estimated using a suitable force field which is used to determine the overall energy of the system [57].
Computer-Aided Drug Design Methods
In silico drug repurposing carvedilol and its metabolites against SARS-CoV-2 infection using molecular docking and ... - Nature.com
In silico drug repurposing carvedilol and its metabolites against SARS-CoV-2 infection using molecular docking and ....
Posted: Mon, 04 Dec 2023 08:00:00 GMT [source]
With increasing library sizes, the computational time and cost of docking itself become the main bottleneck in screening, even with massively parallel cloud computing60. Iterative approaches have been recently suggested to tackle libraries of this size; for example, VirtualFlow used stepwise filtering of the whole library with docking algorithms of increasing accuracy to screen approximately 1.4 billion Enamine REAL compounds23,24. Although improving speed several-fold, the method still requires a fully enumerated library and its computational cost grows linearly with the number of compounds, limiting its applicability in rapidly expanding chemical spaces. The major types of computational approaches to screening a protein target for potential ligands are summarized in Table 2.
Drug targets
The primary objective of CADD is to screen, optimize and evaluate the activity of the compound against the target. It forms the multi-disciplinary approach for both academic and major pharmaceutical companies for better efficacy with no/fewer side effects. Computer-aided drug design (CADD) approaches are playing an increasingly important role in understanding the fundamentals of ligand-receptor interactions and helping medicinal chemists design therapeutics. About 5 years ago, we presented a chapter devoted to an overview of CADD methods and covered typical CADD protocols including structure-based drug design (SBDD) and ligand-based drug design (LBDD) approaches that were frequently used in the antibiotic drug design process.
However, the wider potential of automated chemical design concepts and robotic synthesis in drug discovery remains to be seen. The lack of approved drugs and vaccines for COVID-19 and the high mortality rate of the pandemic necessitate identification of effective therapeutics. With the availability of the complete genome sequence of SARS-CoV-2 [114] and structural elucidation of the viral proteins through X-ray crystallography, NMR spectroscopy, electron microscopy and homology modeling, COVID-19 research has been rapidly pursued.
Got interested in learning about CADD?
Drug Discovery Outsourcing In 2023 — A Recap - Contract Pharma
Drug Discovery Outsourcing In 2023 — A Recap.
Posted: Tue, 02 Jan 2024 08:00:00 GMT [source]
In generative models, synthesizability predictions can be coupled with predictions of potency and other properties towards higher levels of automated chemical design131. Thus, generative adversarial networks combined with reinforcement learning (GAN-RL) were recently used to predict synthetic feasibility, novelty and biological activity of compounds, enabling the iterative cycle of in silico optimization, synthesis and testing of the ligands in vitro50,132. When applied within a set of well-established reactions and pharmacologically explored classes of targets, these approaches already yield useful hits and leads, leading to clinical candidates50,132.
The SARS CoV-2 2′-o-methyltransferase (nsp16) is another important enzyme target essential for viral multiplication. The enzyme precisely protects the viral RNA from the cellular innate immunity by participating in the formation of a specific arrangement known as RNA cap, a structure which contributes to viral RNA stability and effective process of translation [124]. SARS-Cov-2 Nsp13 helicase is one of the critical enzyme among the 16 known CoV Nsp proteins which shows the highest sequence conservation across the CoV family, indicating their importance for viral multiplication.
D, Computational tools are supported by rapid growth of affordable cloud computing, GPU acceleration and specialized chips. A pharmacophore is an assembly (3D arrangement) of 'steric' and 'electronic' features required for optimal supramolecular interaction with a specific biological target structure and to prompt/block its biological response [63]. Ligand-based pharmacophore model generation is based on available information on the biological activities of compounds/ligands. A pharmacophore does not symbolize an actual molecule/ligand or real connection between functional groups, but rather provides an abstract description of molecular features that are vital for molecular interactions between molecules and macromolecular ligands. Molecular descriptors are numerical values used to represent the structural and physicochemical properties of molecules [53, 54]. The molecular descriptor field is strikingly interdisciplinary and includes a number of different theories [55].
Pharmacophore models mapping
"The new algorithm has enormous potential." This is especially true for all medically relevant proteins in the human body that don't interact with any known chemical compounds. The researchers aren't now pursuing these molecules any further with a view to bringing drugs based on them to the market. Schneider says, however, that the algorithm is already being used for similar studies at ETH Zurich and in industry.
The resulting DL model was used to screen the Drug Repurposing Hub database (36) and a known c-Jun N-terminal kinase inhibitor SU3327 was predicted to be an antibiotic targeting E. This molecule is structurally divergent from conventional antibiotics and was confirmed to display bactericidal activity against a wide phylogenetic spectrum of pathogens, demonstrating how ML can guide the antibiotics discovery. To expand the range of structure-based docking applicability to those targets lacking high-resolution structures, it is also tempting to use AI-derived AlphaFold2 (refs. 99,100) or RosettaFold101 3D models, which already show utility in many applications, including protein–protein and protein–peptide docking102. Traditional homology models based on close protein similarity, especially when refined with known ligands103, have been used in small-molecule docking and virtual screening104, therefore AlphaFold2 is expected to further expand the scope of structural modelling and its accuracy.
Since the inherent mutability of the SARS-CoV-2 genome may hinder disease prevention and treatment, CADD can be used efficiently to predict the effects of mutation on drug binding with the molecular receptors [166]. However, CADD methods have some limitations such as lead molecules derived from the virtual screening process that still need validation through preclinical and clinical assessments before market approval [167]. The fact that the molecular mechanism studies underlying the disease pathogenesis of COVID-19 are still underway, and the existence of bias and imbalance in the limited data available can have a major impact on the prediction accuracy of CADD methods such as artificial intelligence [168]. The recent examples of hybrid fragment-based computational design approaches targeting SARS-CoV-2 inhibitors highlight the challenges presented by such targets and allow head-to-head comparisons to ultra-large VLS.
In vitro validation of selected chemical entities demonstrated their inhibitory potentials against ROCK-I and NOX2. Structural information about an identified target is a prerequisite for SBDD, but the structures of several identified neurodegenerative drug targets have yet not been determined [32, 33]. A large number of studies have been conducted using the homology modelling approach to generate structures of identified target molecules. Dhanavade et al. generated the structure of cysteine protease, which degrades amyloid beta peptide, an important causative agent of Alzheimer’s disease [34].
Comments
Post a Comment